RSA Conference was vibrant, alive and full of content in spite of the reported dip in attendance from COVID-19 fears. I had the distinct pleasure to present research and a perspective on AI and Privacy in an advanced session with my longtime friend and colleague Dr. Alon Kaufman of Duality: Ghost in the Machine, reconciling AI and Trust in the Connected World.
Over the course of the last 3 months, Alon and I built up a body of research, taxonomies and perspectives with the goal of making clear the privacy implications of a connected and progressively more adaptive and intelligent world. We began with a belief that existing philosophies on privacy are painfully outdated, specifically that privacy is not limited to a small, squeezed class of data (even in a post GDPR world) and that simply limiting ingestion of that dataset isn’t sufficient for the protection of privacy. We also started from a position that no matter how horribly compromised digital citizens of the world feel today from innumerable breaches, that privacy and personal data are still important.
We started with definitions, because even between us we weren’t 100% straight on jargon and terminology. At least if we put down some guidelines, we could have a clear derivative discussion with the audience and advance topics even if only to disagree on the terms! We set about drawing distinctions in the following ways right off the bat for the big three:
- Data Science (DS): analytics, pattern identification and inference, especially on large data sets
- Machine Learning (ML): algorithms or processes that respond to environmental feedback within boundaries in non-deterministic ways (i.e. not explicit instructions)
- Artificial Intelligence (AI): we mean two things by this in ways that might use either DS or ML
- Pursuit of Human Cognition
- Improvement on a continuum of cognition that becomes more self-aware and able to make rational, cogent inferences or deductions
And while we see tremendous potential in all of these technologies, we were clear about the current state of each as of today (warning, opinions lie ahead!):
- DS: finding meaningful phenomena, not just statistical artifacts. Are we perhaps in a golden age here right now?
- ML: mostly old algorithms being unleashed and now producing results.
- AI: mostly hype. Pursuit of AI has produced results in ML, ironically.
Next up, we discussed the inherent issues in dealing with 1st and 2nd order chaos. 1st order chaos is uncontrollable, much like the weather. Where things get interesting is 2nd order chaos, where the system adapts and an intelligence or “ghost” chooses what to do based on your actions. This in some ways condemns pattern recognition to lag with a shelf-life even in the best of circumstances, until we really create an AI that is as least as smart as us.
We also drew distinctions among ethical, legal and technical frameworks and how they relate to privacy. A recurring theme is the importance of harmony among these:
- Ethics: Privacy: Right now we don’t have a clear, shared ethical framework for privacy, but to be clear a framework here would help determine what is “good.” Examples include:
- Religion: proximity to divinity is what is “good”
- Utilitarianism: most happiness and well-being for most people is “good”
- Negative Consequentialism: least bad outcomes are “good”
- Legality: the law. Period. What is allowed or not allowed according to a given jurisdiction or sovereignty wherever we hang the word privacy.
- Technical: the technical model for understanding privacy and how it does or might work. Remember that this is both descriptive of technology and can also be prescriptive, giving architects the model to use for building future systems.
On the technical front, we started with a graph theory-inspired description of Privacy that I first discussed in a Bloomberg article with another good friend, Joe Moreno. Here is a little of that approach for clarity from our shared article:
Think of “graph theory” as a branch of mathematics that builds models that show pairwise relationships among objects such as people, machines, locations and just about anything else—in effect, a giant Tinkertoy-like structure….the physical world can be thought of as the ultimate graph—that is, that there is an ideal graph that could be built to map out how everything is connected to everything else. The race is on, by the way, for data brokers to develop the most up-to-date, sustainable and useful graphs that get closer to this universal, ultimate graph.
With this basis, we established a 3x3 grid with two dimensions:
- Dimension 1 -- the threat vector enumeration:
- Malicious attacks: this includes adversarial input, tricking with poisonous input; membership inference identifying who is in a training set; and a white box attack as is seen with model inversion attacks.
- Privacy leakage: testing whether the right to be forgotten is possible (which some legislation claims as a right today) and how leakage by inference is possible.
- Ethics and fairness concerns: threats to data autonomy for digital citizens, bias and discrimination from training sets to outputs and the tendency for AI to reinforce existing patterns, and liability models which are essential for modern society (we should never be able to say “the AI is to blame”)
- Dimension 2 -- the machine itself:
- Collecting Data or “input”
- Building a Model
- Productization of the Model or “output”
This lent itself well to a grid of complications:
Table 1: The Problem Taxonomy
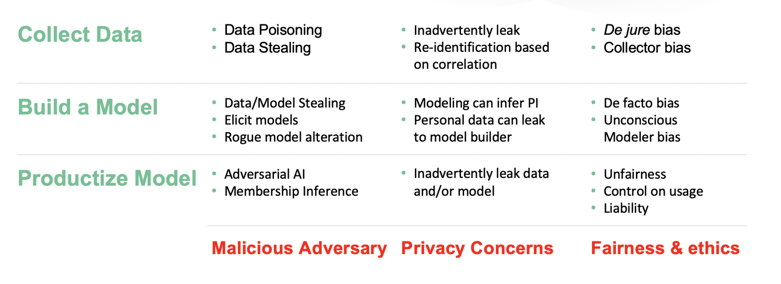
We spent a little time on why the traditional solutions of trusted third parties (for data-in-transit), anonymization (for data-in-use) and classic encryption (for data-at-rest) aren’t up to the task before mapping what solutions exist for each “square” in our problem taxonomy. This leads to not only a solution taxonomy but also a discussion of all the new, emerging and needed Privacy Enhancing Technologies (aka PETs) for the Connected World that we enumerate or envision. PETs encompass a series of technologies built on privacy-by-design principles that protect privacy during the various stages of the data lifecycle.
Table 2: The Solution Taxonomy
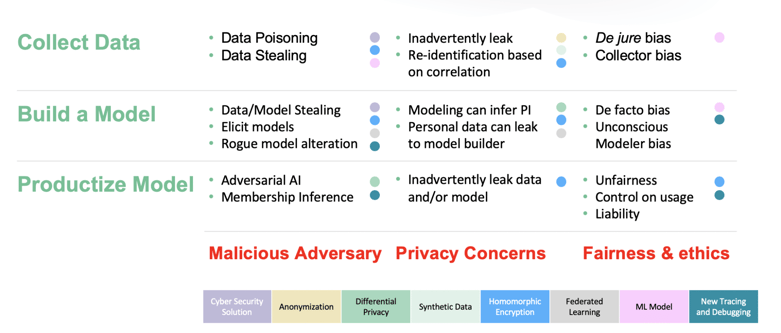
Perhaps most interestingly, we found that ML used to look for patterns in bias was popping up over-and-over as a watchguard technology. This is non-trivial and itself might be a critical service for Watching the Watchers as a service in the future. In other words, we can have watchguard ML and AI to look for the impact of the problem taxonomy.
We also found a new space that needs to be built out. As we did our work for this session, we began to think of this space as a “psychology for AI”, but unlike Human psychology where we can’t directly tap into the infrastructure or code of the brain (yet), we can go far beyond behavioral analysis of AI from the outside. We can get very good at “tracing” and debugging the adaptation and learning of ML and AI. There is a whole new toolkit of technologies to be developed and advanced in this area that we think is a rich potential for future entrepreneurs.
Having said that, we wanted people to leave the session with a sense of what to do, which led to a simple take-away slide after a brief dystopic v. utopic warning:
Table 3: What Can You Do?
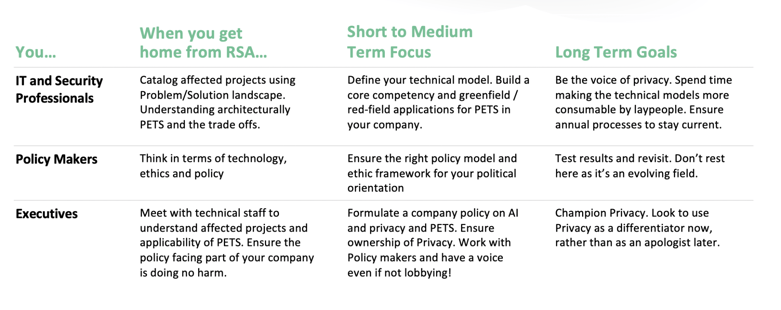
My sincere hope is that this session advanced the discussion of privacy around AI and ML and that people can dive in, take issue with our approach and conclusions and build on it. That’s when it gets fun. So if you want to see a recording of the session, please check it out; and if you have comments or feedback for Alon and I, let us have it!
In case you're on the hunt for even more valuable insights from Sam Curry, we've got a live webinar next Tuesday, March 17th at 11:30 am (EST) | 3:30 pm (GMT) on how to secure business continuity outside of the IT perimeter as we cope with the COVID-19 outbreak.
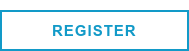